Vision Revolutionized: The Emergence of DINO v2 in Self-Supervised Learning
Written on
Chapter 1: Introduction to DINO v2
The realm of computer vision has undergone remarkable growth in recent years, significantly propelled by advancements in self-supervised learning. This article delves into DINO v2, an advanced self-supervised learning algorithm that elevates the capabilities of computer vision. Developed by Facebook AI, DINO v2 is applicable in various fields, including image classification, object detection, and video comprehension.
We will explore the underlying technology of DINO v2, how it surpasses its predecessor, and the potential consequences of its widespread implementation. Our insights will be enriched by resources such as:
Section 1.1: Understanding DINO v2
DINO v2, which stands for DIstillation of knowledge with No labels and vIsion transformers 2, is a groundbreaking self-supervised learning algorithm from Facebook AI. Building upon the success of the original DINO, it utilizes vision transformers (ViT) to extract insights from images without the need for labeled datasets. DINO v2 enhances its predecessor in multiple aspects, such as improving model efficiency, enabling learning from diverse data types, and achieving better performance in subsequent tasks.
Subsection 1.1.1: How DINO v2 Functions
DINO v2 operates on a teacher-student model to facilitate self-supervised learning. It comprises a "teacher" network and a "student" network, where the student learns from the teacher without any labeled data. Instead, DINO v2 uses contrastive learning to differentiate various instances and features within images.
The success of DINO v2 hinges on its iterative improvement of the teacher network. As the student model learns, the teacher is updated by averaging the parameters from multiple student models. This method enables both networks to enhance their understanding continuously and deliver more accurate feature representations.
DINO v2 presents several advancements compared to its predecessor:
- Multi-modal learning: DINO v2 can learn from different data types, such as images and videos, allowing it to create a more comprehensive understanding of visual content.
- Enhanced efficiency: DINO v2 achieves superior results in fewer iterations, making it faster and more resource-efficient.
- Improved downstream performance: DINO v2 shows better effectiveness in various tasks, including object detection, instance segmentation, and action recognition.
The video titled "DINOV2: Self-Supervised Model for Computer Vision Model Training" provides insights into how this technology is shaping the future of computer vision.
Section 1.2: Applications and Potential Impact
DINO v2 has a wide range of applications across several industries. Here are a few notable examples:
- Medical Imaging: DINO v2 can enhance disease diagnosis and treatment by efficiently analyzing medical images, including X-rays and MRIs.
- Autonomous Vehicles: Its ability to analyze complex visual data makes it ideal for self-driving cars, where real-time decision-making is essential.
- Video Analysis: DINO v2 can improve the processing and analysis of video data for purposes ranging from security monitoring to video editing.
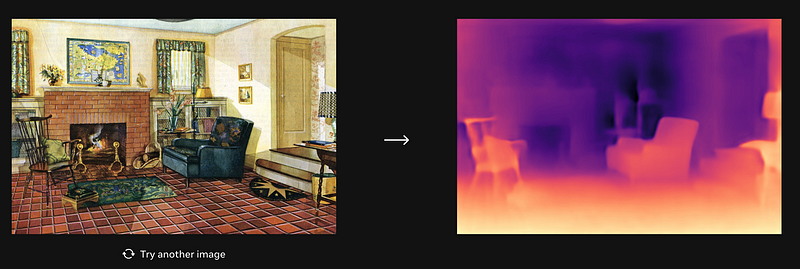
Chapter 2: Conclusion
DINO v2 marks a pivotal advancement in computer vision and self-supervised learning. Its ability to learn from multiple modalities without labeled data opens up exciting opportunities across various sectors. As this technology continues to progress, we can anticipate further innovations and applications, reinforcing the significance of self-supervised learning in the AI landscape.
The second video, "How DINO Learns to See the World - Paper Explained," elaborates on the mechanisms through which DINO v2 interprets visual information.