Understanding AI's Limitations in Text Generation and Image Creation
Written on
Chapter 1: AI Image Creation Capabilities
As I write this, generative AI tools such as DALL-E 2, Stable Diffusion, and Midjourney are advancing rapidly in their ability to produce digital art. These contemporary models can generate a wide array of visual styles, from traditional illustrations to 3D models. Who knows? In the coming weeks, they might even learn to create something entirely novel.
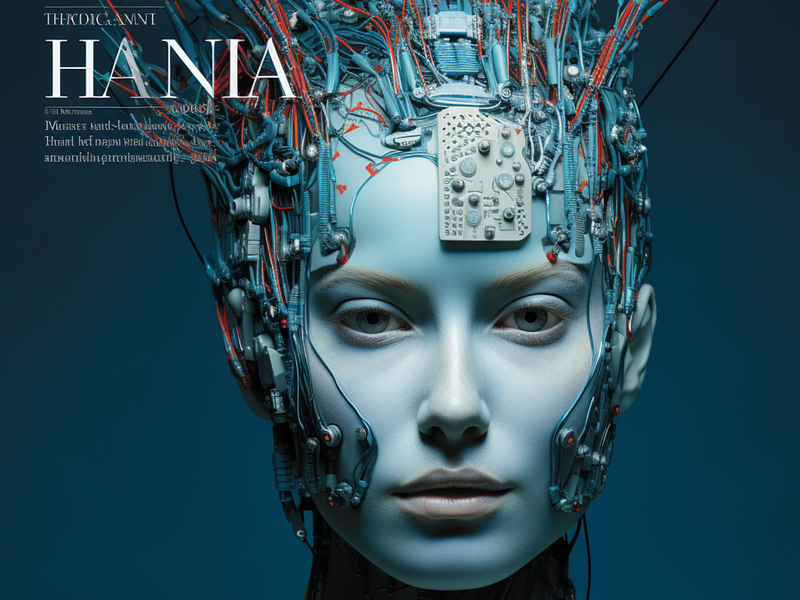
However, challenges remain.
Section 1.1: The Complexity of Human Features
One of the most difficult tasks for AI remains the accurate depiction of human hands. This stems from the datasets used for training; hands are often less prominent in images compared to faces. The same issue applies to other complex features, such as feet and teeth, which results in insufficient training data for the AI to master these intricate details.
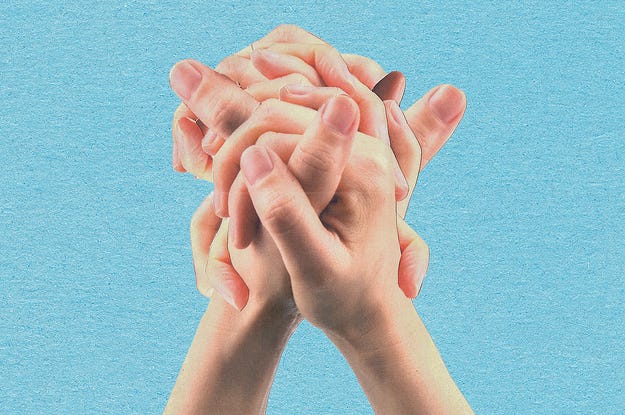
Despite these challenges, each iteration of generative AI shows improvement, which is both exciting and concerning. The potential for AI to create hyper-realistic images poses risks, particularly with the increasing prevalence of deepfakes.
Subsection 1.1.1: Text Generation Challenges
While generative AI excels at producing stunning visuals, it often falls short when it comes to generating coherent text. For instance, despite Midjourney's Versions 4 and 5 being celebrated for their image quality, they struggle to accurately write even simple words like "Hello." Earlier versions were notorious for completely disregarding textual input. Current iterations are better but still misinterpret basic language concepts due to various factors like context, complexity, and ambiguity.
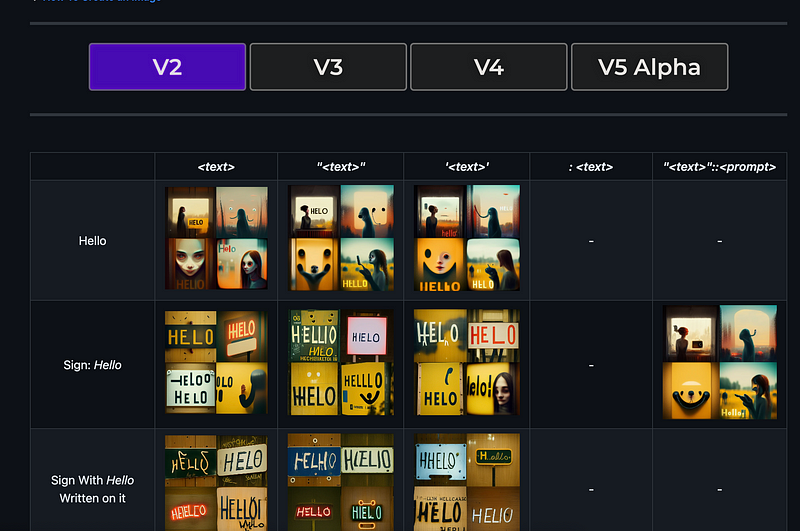
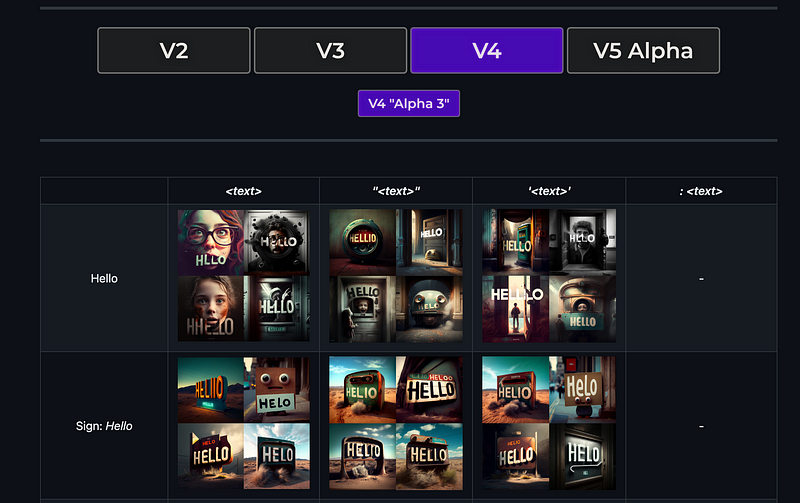
Chapter 2: Insights from Dream Interpretation
Curiously, when people dream, they often perceive nonsensical words and languages. Even if it seems like they are reading, it's usually just a projection of subconscious thoughts. The brain's reading areas are inactive during sleep, leading to the conclusion that genuine reading doesn't occur. However, certain individuals, particularly writers and poets, may experience actual text in their dreams due to their deep engagement with language.
The first video titled "Why AI Image Models Can't Produce Text" delves into the fundamental reasons behind AI's challenges in text generation within images, highlighting issues in training data and model limitations.
Section 2.1: Solutions for Text in AI-Generated Images
When it comes to adding text to AI-generated images, manual editing is often necessary. Basic editing software can remove unwanted symbols and replace them with text. However, advanced techniques like ControlNet, applicable in models such as Stable Diffusion, offer a more sophisticated solution.
ControlNet is a neural network framework that allows users to impose constraints on specific areas shaped like text. By setting these parameters, users can guide the AI to generate text that adheres to desired shapes while maintaining variability in the output.
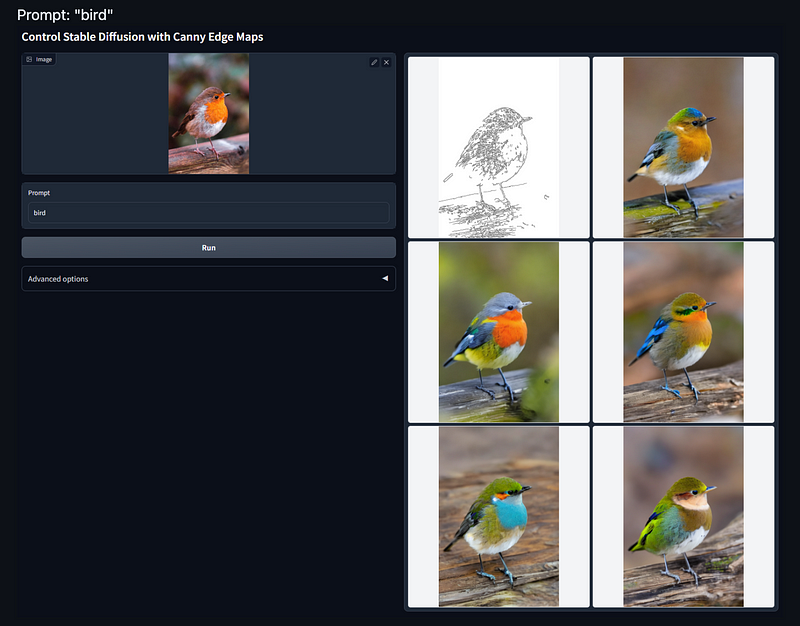
For those interested in exploring this technology further, here's a link to the GitHub repository:
GitHub - lllyasviel/ControlNet: Let us control diffusion models!
Let us control diffusion models! Contribute to lllyasviel/ControlNet development by creating an account on GitHub.
github.com
The second video, "10 Tips for Adding Text to AI-Generated Images," offers practical advice for enhancing the textual aspects of AI-generated artwork, including effective editing techniques and best practices.