Understanding Data Metrics: Why Disputes Are Counterproductive
Written on
Chapter 1: The Foundations of Operationalization
In the early days of psychology, it’s easy to picture academic debates resembling this:
Psychologist 1: “Dear colleague! What you term ‘memory’ is not truly memory! Here’s my definition of memory…”
Psychologist 2: “Dear colleague! I assure you that my definition is indeed memory, while yours is not…”
Imagine them going back and forth like quarreling children. Though I wasn’t present during those formative years (and neither were you), it’s clear that the field experienced enough awkward moments that operationalization became a core concept for every psychology graduate student, myself included, to the point where we might yell “OPERATIONALIZATION” in our sleep.
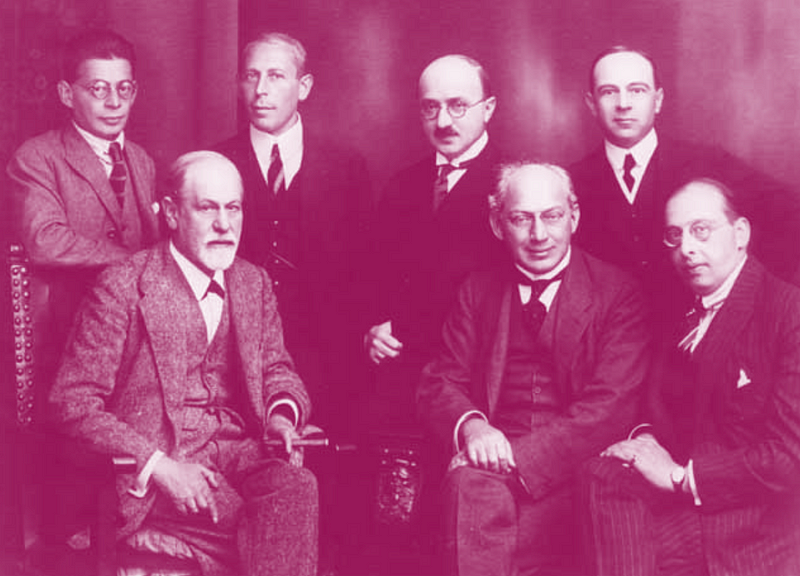
For those unfamiliar with the term operationalization, here’s a brief overview. Essentially, it involves creating measurable proxies to thoroughly explore vague concepts. This practice has been crucial for psychology to transition into a legitimate science, with an essential realization: metrics are not universal but merely useful approximations.
You and I can use the same term with different meanings, provided we clarify our definitions from the start. Just like one can set X=5 and another can set X=10 for different calculations, the academic conversations today are much more polite:
Modern Psychologist: “Dear Colleague! Your interpretation of memory differs from mine. Hence, I cannot incorporate your findings into my research, but I wish you great success in your endeavors!”
Much better, right? The civility brought on by operationalization is indeed refreshing.
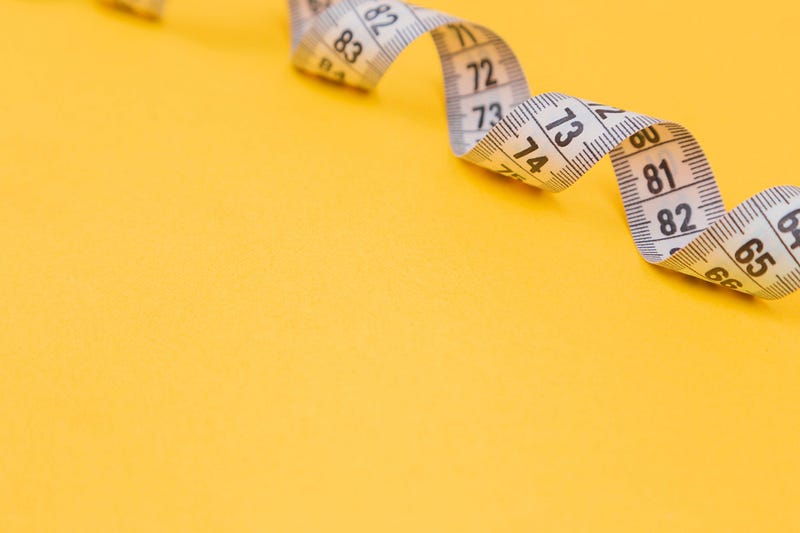
Sadly, the budding field of data science, along with a significant portion of the tech industry, seems to have overlooked the importance of operationalization. Each time I hear an overly enthusiastic newcomer proclaiming, “Dear sir! What you refer to as user happiness is NOT user happiness!!!” I can’t help but feel a mix of amusement and concern.
Getting upset over how others define their terms (I see you, loyal readers who critique my interchangeable use of AI and ML) is not only unhelpful but also stifles human creativity by attempting to confine all meanings into a single box. This box may either become too large to be practical or remain too small, benefiting only a select few who win the initial argument. To maintain its narrowness, the so-called language police resort to harshly criticizing anyone who dares to redefine their cherished terms.
What do you expect people to do—create a metric named UserHappiness1092412? Or perhaps write an entire hyphenated paragraph whenever they wish to articulate a concept?
I advocate for a more relaxed approach. Shorthand and informal language can be beneficial, provided they aren’t misleading. Authors should define their terms when clarity is crucial and adopt a more relaxed tone when it’s less significant.
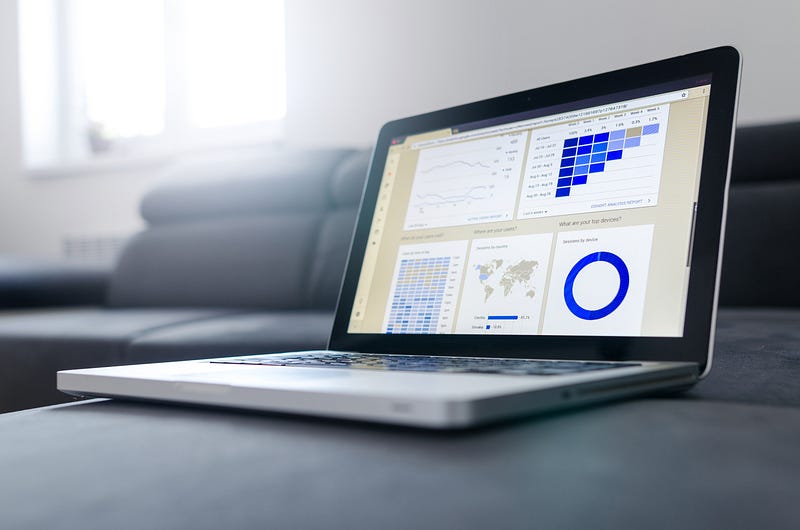
If I begin my next blog entry with, “for this discussion, let’s define AI as airplane,” and then proceed to discuss flying in an AI, I maintain that I’m not in the wrong. Purists might want to reconsider their stance when they realize they engage in similar behavior every time they write code or solve mathematical equations.
The first exposure to operationalization often begins in childhood math classes.
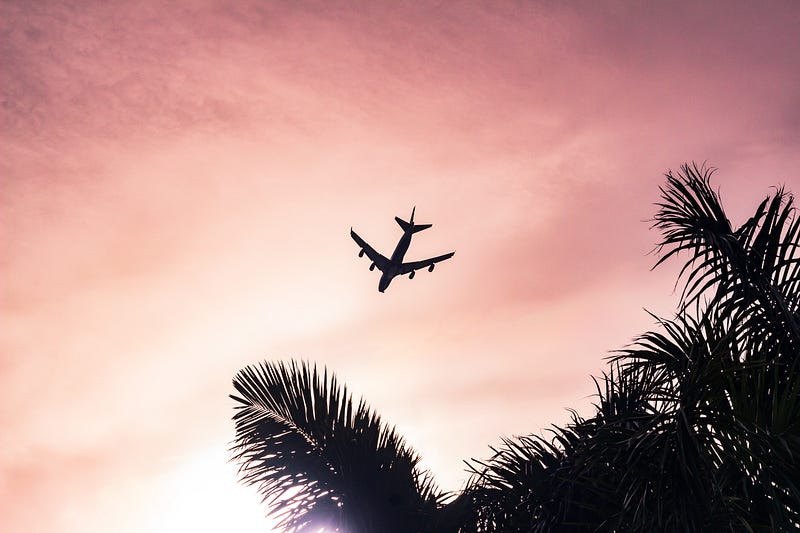
Psychologists and social scientists are trained to recognize that this principle extends to abstract concepts. I only wish this understanding was commonplace. If only more educators emphasized the importance of not overinterpreting abstract terminology without first verifying definitions...
Cultivating the habit of not taking scientific findings at face value until you’ve checked the metric definitions is vital.
If you prefer using “user happiness” to refer to “the likelihood of a user scoring at least 4 stars on this survey,” that’s perfectly acceptable. It’s my responsibility to look up your definition before applying your findings in my work. When offering advice, I should focus on your stated aims rather than my preferences for terminology.
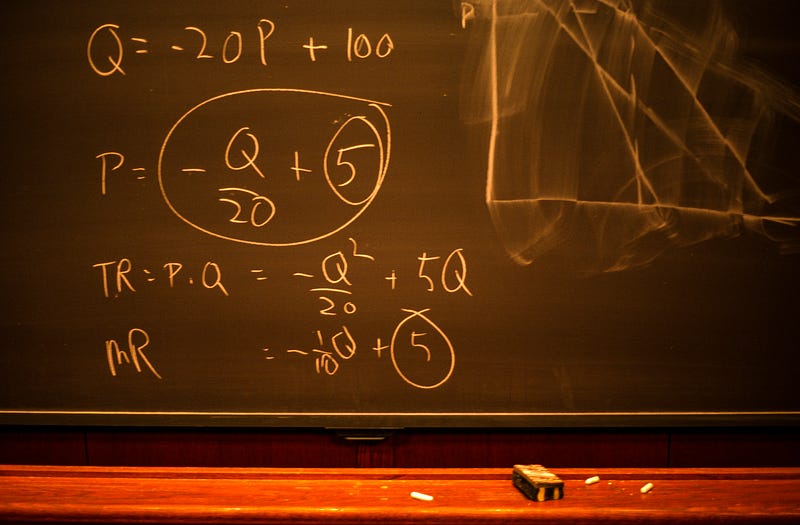
I suspect that improved habits around operationalization could resolve numerous conflicts—imagine if people defined their terms instead of raising their voices.
Even if I disagree with your metric, there’s no need for us to argue about whether something qualifies as user happiness, similar to how we wouldn’t dispute whether the variable I labeled X aligns with the X in your 8th-grade notebook.
If we adopted the mindset that X is merely a placeholder, just like the term “happiness” and all its ambiguous relatives, we would have far fewer disagreements.
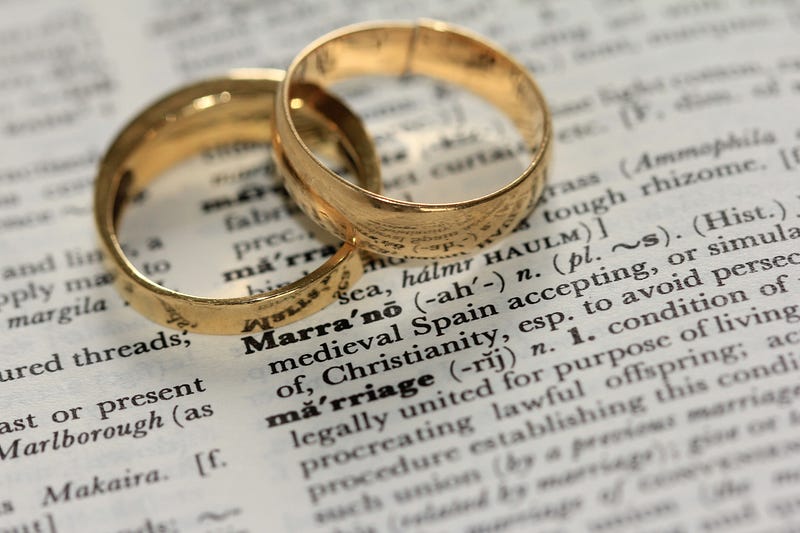
Thanks for reading! Interested in enhancing your skills with a fun YouTube course?
The first video, "Is Backtesting a Waste of Time?", discusses the value and pitfalls of backtesting in data analysis.
The second video, "The Great Conversion Rate Swindle: KPIs, Lies, and Finger Lickin' Deception!", explores the misrepresentation of KPIs and the truth behind conversion rates.
If you enjoyed this piece, feel free to connect with me on Twitter, YouTube, Substack, and LinkedIn. Interested in having me speak at your event? Use this form to get in touch.